How to Avoid Unintended Bias in AI/ML Systems.
After its August 2019 debut of its Apple Credit card, Apple and Goldman Sachs found themselves in an ethical AI/ML nightmare. Users pointed out what seemed to be a gender bias, with women apparently receiving lower credit limits than men. This disparity didn’t go unnoticed where tech luminaries quickly denounced the card’s perceived biases.
Apple’s clarifications only added to the ambiguity. The company’s representatives seemed at a loss to clearly articulate the workings of the card’s algorithm. Meanwhile, Goldman Sachs, responsible for issuing the Apple Card, was quick to dismiss any allegations of gender bias, though their reassurances lacked tangible evidence. Their main defense? An assurance that a third-party had reviewed the algorithm, which, they stressed, doesn’t factor in gender. If gender isn’t considered, where does the disparity stem from?
An algorithm that doesn’t directly consider gender can still exhibit biases against women if it relies on data that indirectly correlates with gender. Numerous studies have highlighted how these inadvertent “proxies” can introduce unintended biases in various algorithms.
As the world continues to embrace the digital age, AI/ML have become integral components of business models, decision-making processes, and innovation drives. However, with the numerous benefits and transformative power of these technologies, come significant ethical concerns. Professionals are at the forefront of navigating these challenges, making it imperative to understand the broader implications of their AI and ML deployments.
AI’s potential for objective decision-making is compromised when tainted with inherent human biases. The true challenge lies not in the technology itself but in ensuring the fairness of the data it processes. A McKinsey study investigating AI bias has presented several key insights:
- The feedback loop from user-generated data can amplify biases. If a society based stereotype is prevalent in the data, it can further cause the bias to be amplified in the output. This bias possibly emerges from user behaviors influencing the algorithm’s recommendations.
- Data collection methods can inadvertently introduce bias. In the realm of criminal justice AI models, focusing on specific locales can intensify surveillance and law enforcement in those regions, reflecting a skewed reality.
- AI models, at times, mirror societal and historical prejudices rooted in their training data. An example is word embeddings (part of Natural Language Processing) absorbing gender biases when trained on news articles.
- Machine Learning systems can recognize patterns that might be socially taboo or illegal. One alarming scenario could be a mortgage lending model associating older age with higher default risks, leading to unlawful age discrimination.
Best Practices to Address Ethical Implications of AI
- Real-World Scenario Testing: Before deploying AI systems, test them using diverse real-world scenarios to simulate actual usage conditions. This can reveal hidden biases that might not be evident in controlled or idealized testing environments. Regularly updating these test scenarios ensures the system remains relevant and unbiased as societal dynamics evolve.
- Test Cases Reflecting Real-World Scenarios for Legal Compliance: Incorporate test cases that simulate a wide range of real-world situations to identify and rectify any illegal biases. Ensure these test cases are constructed in alignment with current laws and regulations. By mimicking true-to-life situations, these test cases can uncover covert biases that might lead to legal violations in actual deployments. Regular reviews and updates to these test cases are essential to maintain compliance as laws evolve.
- Transparency and Explainability: Ensure that AI models can be understood and interpreted by both technical and non-technical stakeholders. An AI system should be able to explain its decisions in clear terms.
- Diverse Training Data: Use a broad and representative dataset that reflects the diversity of the real-world scenario the AI is intended for. This reduces the chance of unintended discriminatory outcomes.
- Ethics Committees: Establish multidisciplinary committees to oversee AI projects. Such committees should include ethicists, sociologists, and representatives from affected communities, ensuring a holistic view of ethical concerns.
- Continuous Learning and Updating: AI models should be periodically updated to reflect the changing dynamics of society, ensuring their relevance and reducing obsolescence-induced biases.
- Stakeholder Participation: Engage users, affected communities, and external experts in the AI development process. Feedback from these groups can provide invaluable insights into potential ethical pitfalls.
- Clear Guidelines and Regulation: Develop in-house guidelines for AI ethics and adhere to external regulations. Encourage the broader AI community to adopt universally accepted ethical standards.
- Accountability Mechanisms: Implement mechanisms to hold systems (and their human developers) accountable for decisions. If an AI system causes harm, there should be clear remediation processes in place.
- Ethical Training: Ensure that those involved in AI development, deployment, and maintenance receive training in ethics, understanding the broader implications of their work.
- Privacy Preservation: Prioritize user privacy by implementing data anonymization, differential privacy, and secure multi-party computation techniques. Ensure AI models respect and protect user data rights.
- Risk Assessment: Before deploying any AI model, conduct a thorough risk assessment to understand and mitigate any potential harmful consequences.
- Open Dialogue: Foster an organizational culture that promotes open discussion about AI’s ethical implications. Encourage teams to raise concerns and propose solutions.
Conclusion:
AI and ML are transformative technologies with enormous potential to change how businesses operate and innovate. However, they also introduce a plethora of ethical challenges that can impact society at large. As executive professionals spearhead the integration of AI and ML into their organizations, a deep understanding of these ethical dimensions is not just recommended—it’s a responsibility. This knowledge will ensure that the deployment of AI and ML aligns not only with organizational goals but also with the broader principles of fairness, transparency, and societal well-being.
latest video
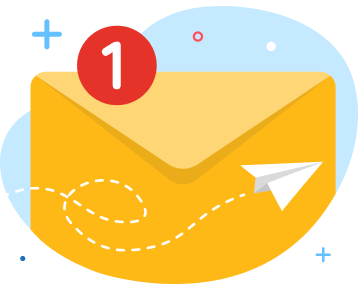
Get Our Newsletter
Never miss an insight!