Get your Ninja On! Understanding the Ninja Tricks Used Against Your AI/ML Systems
It’s no secret the social and security implications of powerful AI capabilities are very concerning for policy makers and businesses alike. While many of these vulnerabilities focus on the worst case scenario somewhere between a Terminator and Matrix style future, the more likely outcomes will be the ability to disrupt business and society through reputation damage, cyber security breaches, and extortion. The adversarial tactics with the current generation of AI/ML tools are more and more prevalent. These so-called “Ninja Tricks” of AI have significant ramifications for organizations seeking to leverage AI’s potential securely.
Textbook Definition
Ninja Tricks also known as Adversarial Attacks refer to: The intentional perturbations made to input data, often imperceptible to humans, designed to deceive AI models into making incorrect decisions or classifications. Such attacks exploit the way AI models, especially neural networks, process information.
Demystifying Ninja Tricks (Adversarial Attacks) through Examples
Imagine a company, Innovators-R-Us, that offers a cloud-based image recognition service. They’ve invested millions of dollars and years of research into training a state-of-the-art deep learning model that can identify thousands of object types in images with unparalleled accuracy. The details of their model, including its architecture and training data, are proprietary and a significant competitive advantage.
A rival company, ShallowPockets Tech, wants to build a similar service but doesn’t want to invest the same time and resources. Instead, they decide to use model extraction to approximate Innovators-R-Us’ model.
Here’s how they might go about it:
- Querying the Model: ShallowPockets Tech starts by submitting thousands of diverse images to Innovators-R-Us service, carefully noting the predictions for each image.
- Creating a Dataset: Using the responses from Innovators-R-Us, ShallowPockets Tech constructs a new dataset. For every image they submitted, they now have a corresponding label (or set of labels) given by Innovators-R-Us’ model.
- Training a Mimic Model: With this newly created dataset, ShallowPockets Tech trains their own deep learning model. Their goal is to make their model’s predictions closely match those of Innovators-R-Us’ model.
- Evaluation: After training, ShallowPockets Tech evaluates their mimic model on a separate set of images, again comparing the predictions with those from Innovators-R-Us’ service. If their model’s performance is close to that of Innovators-R-Us, they’ve successfully extracted or approximated the proprietary model.
While this mimic model might not capture every nuance of the original, it can come surprisingly close, especially if the attacker submits a large and diverse set of queries. This example underscores the importance of considering potential vulnerabilities in AI deployments and the necessity of defense mechanisms against model extraction.
Other examples of how a system can be tricked with unexpected input.
Other examples of how a system can be tricked with unexpected input.
- Disrupting Autonomous Driving Vehicles: By making nearly invisible modifications to road signs, researchers have misled autonomous driving systems. For instance, adding inconspicuous stickers to a stop sign can cause an AI system to misread it as a speed limit sign. https://arstechnica.com/cars/2017/09/hacking-street-signs-with-stickers-could-confuse-self-driving-cars/
- Manipulated Sentiment Analysis: Textual data can be tweaked with certain words or phrases, causing AI sentiment analyzers to interpret neutral or negative comments as positive. https://medium.com/kunumi/manipulating-sentiment-in-nlp-2bbb6351ae3f
- Data Poisoning: Rather than directly altering the input, attackers can subtly manipulate the data used to train the model, ensuring that the AI behaves unpredictably not just for one input but for a whole class of inputs. https://spectrum.ieee.org/ai-cybersecurity-data-poisoning
- Spoofing Face Recognition: There have been instances where attackers used printed patterns on eyeglass frames to trick facial recognition systems. As a result, a person wearing the glasses might be falsely identified as someone else. https://blog.dormakaba.com/the-most-common-facial-recognition-spoofing-methods-and-how-to-prevent-them/
- Tricking Alexa and Siri: AI voice assistants like Amazon’s Alexa or Apple’s Siri can be silently instructed using commands that are altered to be inaudible or unintelligible to humans, yet still recognizable by the AI. This could lead to unauthorized actions, like ordering products online without the user’s knowledge. https://www.nytimes.com/2018/05/10/technology/alexa-siri-hidden-command-audio-attacks.html
- Corrupting Medical Images: Medical diagnostic AI tools can be misled by injecting small amounts of noise into medical images. These alterations might be invisible to the human eye but can cause the AI to miss a tumor or other critical diagnosis. https://link.springer.com/article/10.1007/s43681-021-00049-0
- Sticker Trickery: These are specially crafted stickers or patterns that, when recognized by an AI, can cause it to see something entirely different. For example, placing a certain sticker on a banana can make a neural network think it’s seeing a toaster. https://cset.georgetown.edu/article/hacking-poses-risks-for-artificial-intelligence/
- Malware Manipulation: In cybersecurity, malware can be slightly altered to appear benign to AI-based security systems. This means harmful software can pass through security checks undetected. https://zvelo.com/ai-powered-malware-holds-potential-for-extreme-consequences/
- Cloaking with Patterns: Techniques like wearing certain patterns or using infrared light can cause individuals to become invisible or misidentified by AI-driven surveillance cameras. https://www.hackster.io/news/this-real-life-invisibility-cloak-hides-you-from-person-detecting-machine-learning-models-44fc7c9ee05d
Implications for Decision-Making
- Reputation Damage: A business that falls victim to adversarial attacks can suffer significant reputational damage. Ensuring robust security measures and being transparent about any breaches can help maintain trust with stakeholders.
- Increased Regulatory Compliance: With the rise of adversarial attacks, regulatory bodies may enforce stricter guidelines for AI-driven solutions, especially in critical sectors like healthcare, transportation, and finance. Executives need to stay abreast of these regulations to ensure compliance and avoid potential legal ramifications.
- Investment in Research & Defense: Organizations leveraging AI should consider investing in ongoing research and tools that defend against adversarial attacks. This includes AI models that can detect and resist manipulated inputs.
- Vendor AI Security Evaluation Criteria: When sourcing AI solutions, it becomes imperative to assess vendors based on the resilience of their models to adversarial attacks. This adds another layer to vendor evaluation criteria.
- Specialized Training & Awareness: Organizational training to raise awareness about the nature and risks of adversarial attacks can ensure that all stakeholders, from tech teams to leadership, remain vigilant.
- Strategic Re-evaluation: Adversarial vulnerabilities could necessitate a recalibration of how integral AI is to your core business processes. The degree to which you rely on AI must be weighed against its susceptibility to compromise.
- Increased Financial Costs and Contingency Planning: The financial ramifications of an adversarial attack can be substantial, from immediate operational costs to longer-term reputational losses. Building contingencies in fiscal planning for potential breaches becomes imperative.
- Impact to Consumer Trust & Brand Loyalty: In a consumer-centric world, a single adversarial breach, especially one that compromises user data or safety, can significantly erode trust. Executives must proactively communicate AI’s benefits and security measures to maintain brand loyalty.
- Research and Due Diligence: Where AI assets play a role, due diligence regarding the subjects AI security against adversarial attacks becomes crucial.
- Product & Service Differentiation: Offering AI-driven products or services resilient to adversarial attacks can be a unique selling proposition. It’s not just about the product’s core features but also about its fortified integrity in an increasingly challenging environment.
- Innovation Pipeline Impact: The race to innovate can sometimes bypass rigorous security testing. Leaders must ensure that the pace of innovation does not compromise the robustness of new AI-driven offerings against adversarial attempts.
- Cultural Values & Ethos: Beyond technical defenses, creating an organizational culture of vigilance, responsibility, and continuous learning can be a bulwark against the human errors that often accompany security breaches.
- Cross-Industry/ Cross-Governmental Collaboration: Adversarial attacks aren’t confined to one industry. Collaborating across sectors can facilitate shared learnings, best practices, and even collective investments in research and defenses.
- Geopolitical Considerations: The sources of adversarial attacks can often be international, bringing into play geopolitical considerations and necessitating a global response strategy.
- Ethical and Social Responsibilities: If a company’s AI is vulnerable, it could be used in misleading or harmful ways, making the company complicit, even if unintentionally. Executives must ponder the broader societal implications and their company’s ethical stance.
Adversarial attacks, or the “Ninja Tricks” of AI, illuminate the vulnerabilities inherent in AI systems. For the savvy leader, understanding these potential pitfalls is not about inducing fear but fostering preparedness. By recognizing the risks and implementing proactive measures, organizations can harness the power of AI while minimizing vulnerabilities. The future will inevitably see a cat-and-mouse game between AI advancements and adversarial techniques, and staying informed will be the organizations strongest defense.
latest video
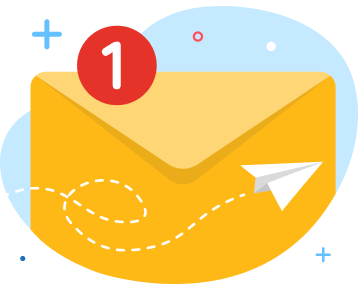
Get Our Newsletter
Never miss an insight!