Is Your AI Model Doomed to Fail?
The rapid evolution of artificial intelligence and machine learning (AI/ML) has ushered in a new era of technology, from personal assistants in our pockets to advanced data analytics informing business decisions. But the true powerhouse behind successful AI models isn’t necessarily the algorithms—it’s the data. In this exploration, we’ll delve into the importance, intricacies, and challenges of testing data in AI/ML.
Textbook Definition: Testing data in the context of machine learning is a subset of data that’s used to evaluate the performance of a trained model. Unlike training data, which shapes the model, testing data is kept separate to offer an unbiased assessment of a model’s performance in scenarios it hasn’t previously encountered. Testing data is not used during the training phase.
Why is Testing Data so Integral to AI/ML?
Imagine constructing a state-of-the-art vehicle, and then assessing its capabilities by only driving it around your backyard. The results will hardly reflect how the car would perform on a highway or up a steep mountain road. Similarly, without testing our AI/ML models on diverse, unseen data, we cannot gauge their accuracy or predict how they will behave in real-world situations.
The old programming adage “garbage in, garbage out” resonates deeply in AI/ML. Without appropriate testing data, even the most sophisticated models can become functionally irrelevant, or worse, erroneous. Consider testing data as the final examination a model must take after its extensive learning from training data. Only through this rigorous testing can we trust a model’s predictions and actions in diverse, real-world situations.
The Challenges Encountered in Sourcing and Utilizing Testing Data:
- Representation and Bias: For a model to be universally effective, its testing data must encompass a vast array of scenarios, environments, and edge cases. A classic example of this challenge is seen in facial recognition technologies. When tested on a non-representative dataset, predominantly containing images of individuals from one demographic, these systems have shown biases, often leading to inaccuracies when presented with faces from underrepresented demographics.
- Data Scarcity: Certain applications, especially niche or emerging ones, suffer from a lack of abundant data. Think of medical AI systems designed to diagnose extremely rare diseases. Here, limited data might make a model appear effective, but its reliability remains questionable until tested on a diverse set of cases.
- Mismatched Data Distributions: At times, testing data might arise from a source or environment different from the training data. For instance, a model trained to understand speech from high-quality, noise-free recordings might struggle when tested on real-world, noisy data.
- Temporal Dynamics: The world changes, and with it, data distributions can shift. A model trained and tested on data from a specific time period may not necessarily be relevant in the future. For example, customer preferences or market dynamics from a decade ago may not accurately reflect today’s realities.
Case Studies Illuminating the Complexities:
- Healthcare: An AI system developed to assist radiologists in detecting tumors was trained using images from high-resolution MRI machines. However, during testing, when images from older, less precise machines were introduced, the system’s performance deteriorated. This highlighted the significance of ensuring testing data diversity across equipment variations.
- Finance: In the world of algorithmic trading, a model might be trained on bullish market conditions. When tested only on similar data, its performance might seem stellar. But, in the unpredictable world of finance, the true test of such a model lies in its ability to adapt to bearish markets or financial crises.
To Put it in Simpler Terms:
Imagine a chef who has trained exclusively with high-quality, organic ingredients. If suddenly presented with lesser quality ingredients, the outcome of their dish remains uncertain. The dish might still be palatable, but it might not meet the standards set during the training phase.
Or better yet, picture a student who’s only been tested on algebra and excels in it. However, if presented with a calculus problem, their performance remains uncertain. They might be an A+ algebra student but might not fare as well in calculus.
The Way Forward: Best Practices and Considerations:
- Training Data is not Test Data: To ensure an impartial evaluation of your AI/ML model, it’s critical to isolate your testing data from your training data. This separation allows the model to be assessed in unfamiliar scenarios, thereby providing a more comprehensive understanding of its performance capabilities. In essence, reserve a distinct subset of data exclusively for testing purposes and do not incorporate it during the model’s training phase.
- Diverse Data Collection: Strive to source data that captures a broad spectrum of scenarios. This might involve collaborations, partnerships, or even crowd-sourcing.
- Continuous Model Evaluation: The testing phase isn’t a one-off. Regularly re-evaluate models using fresh, updated testing data to ensure their continued relevance.
- Transparency and Ethical Considerations: As AI/ML practitioners, it’s imperative to be transparent about the limitations of a model based on its testing data. Also, ethical considerations, especially in terms of data privacy and bias mitigation, should be at the forefront of any testing strategy.
- Work to identify bias using test data in AI/ML: Proactively engage in bias identification by utilizing your test data within AI/ML frameworks. Allocate specific subsets of your test data to evaluate the model across diverse scenarios and demographics. This approach helps in uncovering latent biases and ensures that the model’s performance is equitable and representative.
In Conclusion:
The nuances of testing data in AI/ML cannot be overstated. As the AI landscape continues to evolve, our strategies around data collection, testing, and model evaluation need to be agile, ethical, and robust. By understanding and addressing the challenges of testing data, we not only pave the way for more reliable AI systems but also foster trust and credibility in this transformative technology.
latest video
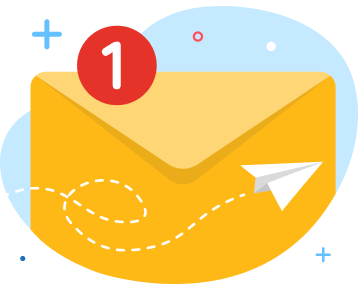
Get Our Newsletter
Never miss an insight!