A Second Chance at Credit: AI’s New Role in Banking
For years, Emma Lopez, a fictionalized freelance graphic designer, felt the weight of an imperfect credit score. Traditional banks had denied her loan applications, citing her irregular income and limited credit history. But the emergence of machine learning in banking changed her story, and many others’, by offering a more nuanced understanding of creditworthiness. This is the tale of how cutting-edge technology gave Emma a chance to prove her financial reliability in ways that were never before possible.
The Problem with Traditional Credit Assessments:
Like Emma, many individuals and small business owners face barriers when applying for credit through conventional banking channels. These systems often fail to recognize the financial stability and potential of applicants whose records don’t fit the traditional mold. The one-size-fits-all approach has kept many deserving borrowers on the sidelines.
Machine Learning: A New Frontier for Financial Fairness:
Machine learning (ML) stands to revolutionize this antiquated process by taking into account a myriad of factors beyond just credit scores and fixed incomes, from spending habits to savings goals and even educational background.
Emma’s Story: A Glimpse into the Future of Credit Applications:
When Emma applied for a personal loan to expand her business, she was no longer constrained by the shackles of outdated credit scoring. The bank’s ML algorithm assessed her entire financial narrative — her consistent contract work, her diligent saving habits, and her entrepreneurial spirit. It was a holistic approach that saw potential where traditional systems saw risk.
The Advantages of Machine Learning in Credit Applications:
- Comprehensive Evaluation:
- For people like Emma, ML algorithms consider diverse data, painting a fuller picture of an applicant’s financial health.
- Predictive Analytics:
- Beyond present circumstances, ML can forecast future income potential and spending behaviors, providing a dynamic assessment of creditworthiness.
- Automated Decisions:
- Speed is of the essence in financial matters. ML streamlines the application process, providing quick and often more favorable outcomes.
Real-World Impact and Implementation:
Emma’s successful loan application is not an isolated case. Many banks and financial institutions are leveraging ML to diversify their portfolios and embrace customers who are financially responsible yet have been previously marginalized.
Challenges Ahead:
Adopting ML isn’t without its challenges. Concerns around data privacy, algorithmic bias, and regulatory compliance must be diligently addressed. But the potential benefits — increased inclusivity, better risk management, and enhanced customer experience — are too significant to ignore.
The Future is Inclusive:
Emma’s story embodies the future of credit applications — a future where machine learning opens doors for hardworking individuals who have been historically overlooked by the credit system. With ML, banks can not only expand their customer base but also contribute to a more equitable financial landscape.
Conclusion:
Machine learning in credit applications isn’t just about technology; it’s about people like Emma who deserve a chance to realize their dreams. As banks continue to integrate these advanced analytical tools, they are not only changing the narrative for individuals but also transforming the industry as a whole. With each approved application, ML is helping to build a more financially inclusive world, one deserving borrower at a time.
latest video
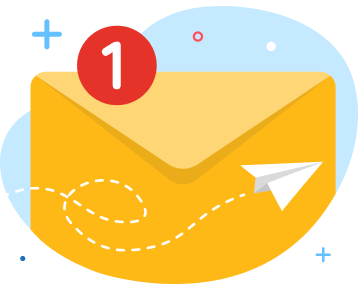
Get Our Newsletter
Never miss an insight!