Opportunities for the US Treasury to Lead AI Practically
I recently reviewed the U.S. Department of the Treasury’s report “Managing Artificial Intelligence-Specific Cybersecurity Risks in the Financial Services Sector.” First things first, as a former IRS executive, these opinions are solely my own and are not in any capacity to be considered an opinion from the treasury or IRS. Now that that is out of the way, the report specifically addresses the emerging challenges and opportunities associated with the integration of AI technologies in financial services. In response to Executive Order 14110, the report focuses on identifying and mitigating AI-related cybersecurity and fraud risks through comprehensive analysis and recommendations. Drawing from 42 in-depth interviews with industry stakeholders, the report highlights current AI use cases, emerging threats, and best practices for risk management within financial institutions.
The report underscores the dual nature of AI in financial services: while AI enhances operational efficiency and fraud detection capabilities, it also introduces new vulnerabilities. Financial institutions have integrated AI into various operations, particularly in cybersecurity and anti-fraud efforts. However, existing risk management frameworks may not be sufficient to address the unique challenges posed by advanced AI technologies such as Generative AI. The report advocates for a cautious and collaborative approach, emphasizing the need for cross-enterprise collaboration and the development of tailored AI risk management strategies.
In addition to detailing the current landscape, the report provides some actionable best practices for financial institutions. These include enhancing data privacy and security, fostering industry-wide collaboration for fraud data sharing, and ensuring regulatory compliance through robust governance frameworks. The Treasury’s findings highlight the importance of adapting traditional risk management practices to address the complexities of AI, ultimately aiming to bolster the resilience and security of the financial services sector in the face of rapid technological advancements.
Recommendations for the Treasury
While the Treasury’s report provides a solid foundation, there are several opportunities for improvement. The Treasury needs to delve deeper into shifting the mindset of how to address AI challenges. Past frameworks are notoriously slow in the government for dealing with risk management. Additionally, expertise in AI is scarce, with true AI experts commanding seven-figure salaries due to high demand and limited supply. Although using existing frameworks is a good starting point, these frameworks alone are not sufficient to address AI’s unique challenges. AI is fundamentally different and evolves too quickly for traditional IT management approaches, and we as IT leaders are still on a steep learning curve. Recognizing this, the Treasury must take a more forward-thinking approach to help financial institutions, especially smaller ones (read most financial institutions), navigate the complexities of AI.
Hubris may make us think we can manage this like anything else IT, but ignores the realities of a true ground changing technology revolution. This is the equivalent of going from horse and buggy transportation to space travel.
To expand upon the limited practical guidance provided in the Treasury AI report, here are several areas where the Treasury could offer more detailed, actionable steps for financial institutions to implement the report’s recommendations effectively:
- Developing AI Risk Management Frameworks
- Detailed Framework Blueprints: Provide blueprints for AI-specific risk management frameworks, including sample policies, procedures, and controls tailored to different types of AI applications.
- Step-by-Step Implementation Guides: Offer step-by-step guides on how to integrate AI risk management into existing enterprise risk management (ERM) systems, including timelines, milestones, and key performance indicators (KPIs).
- Mitigating AI Bias and Ethical Concerns
- Bias Mitigation Techniques: Detail specific techniques for identifying and mitigating biases in AI models, such as diverse data sourcing, fairness testing, and bias correction algorithms.
- Ethical AI Checklists: Provide checklists for ensuring ethical AI use, covering topics like transparency, accountability, and fairness. Include examples of ethical dilemmas and how to address them.
- Enhancing Data Privacy and Security
- Data Protection Protocols: Outline robust data protection protocols, including encryption, anonymization, and access controls specifically designed for AI training and operational data.
- Privacy Impact Assessments (PIAs): Offer templates and guides for conducting PIAs to evaluate the privacy risks associated with AI systems and how to mitigate them.
- Supporting Smaller Institutions
- Scalable AI Solutions: Suggest scalable AI solutions that smaller institutions can adopt without significant resource investment, such as cloud-based AI services and open-source tools.
- Collaborative Platforms: Recommend collaborative platforms or consortia where smaller institutions can share resources, data, and expertise to enhance their AI capabilities.
- Regulatory Compliance and Best Practices
- Compliance Roadmaps: Provide roadmaps for compliance with existing and emerging regulations related to AI, including specific steps to align with regulatory expectations.
- Audit and Assurance Checklists: Create detailed checklists for internal audits and assurance processes to ensure AI systems comply with regulatory requirements and best practices.
- AI Model Development and Validation
- Model Validation Protocols: Share detailed protocols for validating AI models, including best practices for testing, monitoring, and retraining models to ensure they remain accurate and reliable.
- Scenario Planning: Include examples of scenario planning exercises to test AI systems under various conditions and identify potential failure points.
- Fraud Detection and Prevention
- Collaborative Data Sharing Frameworks: Offer practical frameworks for secure and compliant data sharing among financial institutions to improve fraud detection and prevention capabilities.
- Case Studies: Provide case studies of successful AI implementations in fraud detection, highlighting challenges faced, solutions implemented, and outcomes achieved.
- Cybersecurity Enhancements
- AI-Specific Cybersecurity Controls: Detail specific cybersecurity controls for AI systems, such as securing AI training data, protecting AI models from adversarial attacks, and monitoring AI outputs for anomalies.
- Incident Response Plans: Offer templates for AI-specific incident response plans that outline how to detect, respond to, and recover from AI-related cybersecurity incidents.
- Training and Skill Development
- Training Programs: Suggest comprehensive training programs for staff on AI technologies, risk management, and cybersecurity, including online courses, workshops, and certification programs.
- Skill Development Pathways: Provide pathways for developing AI expertise within financial institutions, including recommended qualifications, certifications, and career development opportunities.
- Vendor Management
- Vendor Evaluation Criteria: Detail criteria for evaluating AI vendors, including questions to ask about data security, model transparency, and compliance with ethical standards.
- Contractual Safeguards: Offer sample contractual clauses to ensure vendors meet specific AI risk management and cybersecurity requirements.
By incorporating these detailed, actionable steps, the Treasury can better equip financial institutions to manage AI-specific risks effectively and leverage AI technologies responsibly and safely.
latest video
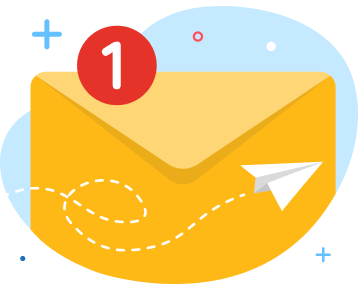
Get Our Newsletter
Never miss an insight!