Predictive Maintenance – How Something Routine Became Very Exciting
Traditionally, manufacturers relied on scheduled maintenance routines or reactive approaches, addressing equipment issues only after a failure occurred. Predictive maintenance, facilitated by AI, represents a significant leap from traditional maintenance practices. AI has shifted this paradigm by enabling a predictive approach, where data-driven insights allow manufacturers to anticipate and prevent equipment failures before they disrupt production.
The cornerstone of AI in predictive maintenance is its ability to analyze vast quantities of data from machinery sensors. These sensors, embedded in manufacturing equipment, continuously collect data on various parameters such as temperature, vibration, and sound. AI algorithms process this data in real-time, identifying patterns and anomalies that are indicative of potential equipment issues. This early detection is crucial in preempting breakdowns and scheduling maintenance activities without hampering production schedules.
Another significant advantage of AI-driven predictive maintenance is cost savings. Unscheduled downtime in manufacturing can be extremely costly, not just in terms of repair expenses but also due to lost production time and delayed shipments. By accurately predicting equipment malfunctions, AI allows manufacturers to minimize downtime, plan maintenance activities during non-peak hours, and manage resources more effectively. This proactive approach can lead to substantial cost reductions and improved bottom-line performance.
AI in predictive maintenance also plays a pivotal role in extending the lifespan of manufacturing equipment. By identifying and addressing maintenance needs early, AI helps prevent minor issues from escalating into major failures that could permanently damage equipment. This not only saves on expensive replacement costs but also maximizes the return on investment in machinery.
The integration of AI in predictive maintenance is also fostering a culture of continuous improvement in manufacturing operations. Data collected and analyzed by AI systems offer valuable insights into equipment performance and operational inefficiencies. Manufacturers can use these insights to refine their processes, enhance product quality, and optimize energy usage, contributing to overall operational excellence.
However, the implementation of AI in predictive maintenance is not without its challenges. One of the primary hurdles is the integration of AI with existing manufacturing systems and infrastructure. Many manufacturers operate on a mix of old and new equipment, and ensuring compatibility between these and advanced AI systems can be complex. Additionally, the successful deployment of AI-driven predictive maintenance requires a skilled workforce capable of interpreting AI insights and making informed maintenance decisions.
Looking ahead, the advancements in AI-driven predictive maintenance are set to accelerate further, fueled by continuous advancements in AI algorithms, sensor technology, and data analytics. As manufacturers increasingly embrace digital transformation, predictive maintenance powered by AI will become an integral part of modern manufacturing ecosystems. This shift not only promises enhanced operational efficiency and cost savings but also positions manufacturers to be more responsive and adaptive in an ever-evolving market landscape.
latest video
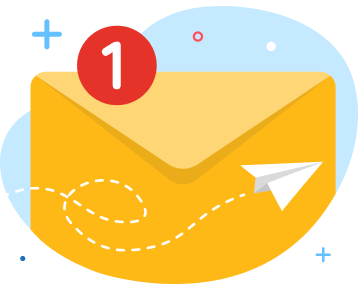
Get Our Newsletter
Never miss an insight!